The healthcare sector is continuously developing, influenced by technological advancements, demographic changes, and the evolving needs of patients: innovation, sustainability, integration of social care, cost management, and workforce adaptation are increasingly highlighted as key trends in the sector, at local, European, and global levels.
The integration of Artificial Intelligence (AI) into medical practice is transforming the way healthcare professionals diagnose and treat patients, enhancing the quality of care and optimizing clinical processes.
Recent reports from the World Health Organization (WHO) and the OECD – Organisation for Economic Co-operation and Development – emphasize that AI can improve access to services, address shortages of qualified personnel, and reduce healthcare system costs. Its integration is seen as a key to tackling public health challenges, such as pandemics and chronic diseases.
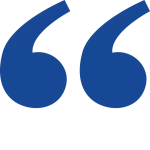
The adoption of AI could transform global health by providing advanced tools for surveillance, drug development, diagnosis, treatment, and patient management.
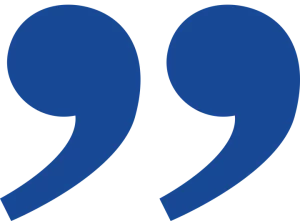
On the other hand, as Dr. Riccardo Lucis – Medical Doctor (MD) of S.C. Microbiology and Virology – Friuli Occidentale Health Authority (ASFO) – coordinator of the working group on Artificial Intelligence of AMCLI – Italian Clinical Microbiologists Association – states: ‘AI is a branch of computer science that is finding renewed global interest due to the explosion of so-called generative AI (e.g., ChatGPT). AI has long been embedded in our daily lives: think of our smartphones, voice recognition, search suggestions, or autocomplete of sentences or words.’ He continues, ‘In particular, some branches of AI, such as Machine Learning (ML) and Deep Learning (DL), focus on using algorithms and mathematical models to teach computers how to analyze data and make decisions by defining patterns and schemes. These models have been used in laboratories since the days of MYCIN, a computer program developed by Stanford University in the 1970s, based on rules drafted by expert physicians and scientific literature, enabling the emulation of a human expert’s decision-making process. In our daily lives, we use these technologies for automatic cell recognition (in hematology and pathology labs), categorizing urine and stool samples (analysis and microbiology labs), or identifying specific patterns of antibiotic resistance (for example, the expert systems of various commercial software, based on EUCAST and/or CLSI guidelines) that escape the human eye, both when studying rare diseases and when analyzing vast amounts of data, in quantities impossible for the human eye alone (interpretations of Next-Generation Sequencing – NGS technologies). It is important to emphasize that the role of humans remains essential to supervise and validate the choices made by these algorithms.’
Dr. Andrea Padoan, who leads the AI Working Group of EFLM – European Federation of Clinical Chemistry and Laboratory Medicine – and is an Associate Professor of Clinical Biochemistry and Clinical Molecular Biology at the University of Padua, highlights some concrete applications of AI in Laboratory Medicine through ‘the use of digital systems for image analysis in hematological samples, through which blood cells are automatically classified, anomalies are identified more quickly, reducing analysis times and, ultimately, human error. Another series of applications involves the technological enhancement of diagnostic systems, leading to a reduction in their size and the associated energy costs. In some cases, AI algorithms can effectively replace cumbersome electronic components, miniaturizing systems and making them increasingly connected and secure.
Another area where AI may demonstrate significant impact is the ability to predict diseases and personalized medicine, allowing doctors to intervene preventively or tailor treatment based on individual risk profiles.
Among the most ambitious projects for AI in laboratory medicine are improving operational efficiency, optimizing workflows, and creating systems for advanced monitoring of sample transportation and storage, as well as clinical decision support systems that assist laboratory professionals in many areas, improving the accuracy, safety, and effectiveness of clinical decisions.’
In a reality like the European Institute of Oncology (IEO) in Milan, the use of AI already plays a crucial role through the Clinical Data Platform (IEO CDP).
‘This platform acts as a health data lake, fueled by clinical and (multi)omic data, and offers integrated tools to develop AI models, including those for generative AI, to extract useful clinical information from reports. Through dashboards and web apps in Google Cloud, the data is made available for clinical monitoring and research. Furthermore, the Value Based Medicine platform (IEO VBM) measures patient-reported outcomes (PROMS) and determines the likelihood of postoperative complications using AI models, contributing to a more personalized and predictive approach to patient care.’
Challenges and Future Perspectives
Despite the benefits, the adoption of AI in medicine presents significant challenges, such as ethical issues, data privacy, and the need for training for healthcare professionals.
‘Even back then [MYCIN in the 1970s], some concerns arose: acceptance by professionals of this system and worries about legal responsibility. […] Unlike the technological limitations of that era, now, thanks to the development of the internet and high computing power and storage, we have more complex systems.
It is important to emphasize that the role of humans remains essential to supervise and validate the choices made by these algorithms.’ Dr. Riccardo Lucis
‘Despite the opportunities presented, there are also significant challenges to overcome to achieve effective integration of AI in Laboratory Medicine. One of the main difficulties is the need for high-quality data to train AI algorithms. Laboratory data can vary significantly between laboratories, geographical regions, and patients, making it challenging to create generalizable AI models. Moreover, the integration of AI raises ethical questions, such as diagnostic responsibility and the protection of clinical data privacy.’ Dr. Andrea Padoan
The WHO and OECD are working to develop regulations and ethical standards to ensure that the use of AI occurs safely and responsibly.
For more details, you can consult the official sources: